On March 7, the next Swiss referendum vote will be held, with votes on a ban for full face coverings, the e-ID Act, and the economic partnership agreement with Indonesia. As this national vote approaches, the EPFL election prediction tool, Predikon, is rolling out some improvements with new features.
Predikon is a tool based on probabilistic modelling, large-scale data analytics and machine learning. The aim of Predikon is to better predict final election and referendum results from partial ballot counts. This tool was developed by a team of researchers at EPFL’s Laboratory for Information and Network Dynamics (INDY), in particular by PhD student Victor Kristof, former EPFL Master student Alexander Immer, and former PhD student Julien Herzen, under the supervision of Prof. Matthias Grossglauser and Prof. Patrick Thiran.
Since the last referendum in November, the Predikon team set three development objectives for their tool. First, to predict the number of cantons accepting a vote to account for the double majority, i.e., the majority of the cantons as well as the overall majority. This double majority rarely affects the referendum results but it was, to the surprise of many, that the initiative for responsible businesses was rejected in November as a result of lack of canton majority. Taking into account the majority of cantons is thus one of Predikon’s new features for the upcoming referendum.
The second objective was to include a margin of error for the predictions. For this, they have decided to develop and test two separate approaches, The first through Monte Carlo simulation and the second by using Bayesian inference.
A Monte Carlo simulation is a technique used to estimate the probability of different outcomes. For this, they use the partial results to simulate the outcome of a vote 10,000 times and average these simulations to obtain a point-wise prediction.The variance of the 10,000 outcomes gives a margin of error. The figure below shows a visualisation of the margin of error using the Monte Carlo simulation.
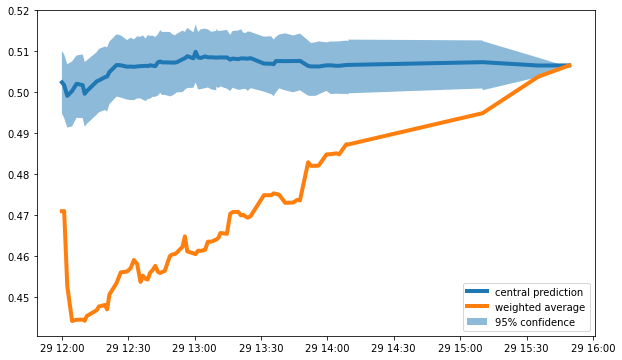
The Bayesian inference approach consists in learning a probability distribution for the final outcome, i.e., a range of possible values with different probabilities of occuring, Hence, this yields a prediction that is a distribution of values rather than a single value. The mean of this predicted distribution gives a point-wise prediction and the variance gives the margin of error. This second approach is the one that will be used by Predikon for their next results as they found it to be both more accurate and computationally more efficient. The figure below shows a visualisation of the margin of error and the prediction using this technique.
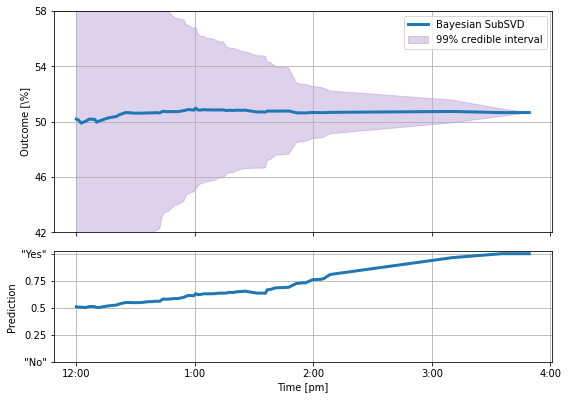
Currently, Predikon predicts the national results within the first minute of the ballot counting. The last objective would be to make accurate predictions before the day of the vote. For now, their idea is to collect their own survey data from the Predikon website. Users can help Predikon’s predictions by indicating their voting intentions and municipality of residence under the corresponding vote section, so if you are planning on voting on March 7, this is a great way to contribute to the development of Predikon!
We are following the evolution of the project until the next vote, which will take place on March 7. An article every month will enable you to observe the evolution of the project, (almost) live, accompanying Victor Kristof and his collaborators in the development of Predikon.
This article is the second in a series about Predikon. Read the rest of the story here:
- A Journey With Predikon (1/3)
- > A Journey With Predikon (2/3)
- A Journey With Predikon (3/3)